Alina Oprea is a Professor at Northeastern University in the Khoury College of Computer Sciences. She joined Northeastern University in Fall 2016 after spending 9 years as a research scientist at RSA Laboratories. Her research interests in cyber security are broad, with a focus on AI security and privacy, ML-based threat detection, cloud security, and applied cryptography. She is the recipient of the Technology Review TR35 award for her research in cloud security in 2011, the Google Security and Privacy Award in 2019, the Ruth and Joel Spira Award for Excellence in Teaching in 2020, and the CMU Cylab Distinguished Alumni Award 2024. Alina served as Program Committee co-chair of the flagship cyber security conference, the IEEE Security and Privacy Symposium in 2020 and 2021. She also served as Associate Editor of the ACM Transactions of Privacy and Security (TOPS) journal and the IEEE Security and Privacy Magazine. Her work was recognized with Best Paper Awards at NDSS 2005, AISEC in 2017, and GameSec in 2019.
About AdvML-Frontiers'24
Adversarial machine learning (AdvML), a discipline that delves into the interaction of machine learning (ML) with ‘adversarial’ elements, has embarked on a new era propelled by the ever-expanding capabilities of artificial intelligence (AI). This momentum has been fueled by recent technological breakthroughs in large multimodal models (LMMs), particularly those designed for vision and language applications. The 3rd AdvML-Frontiers workshop at NeurIPS’24 continues the success of its predecessors, AdvML-Frontiers’22-23, by delving into the dynamic intersection of AdvML and LMMs.
The rapid evolution of LMMs presents both new challenges and opportunities for AdvML, which can be distilled into two primary categories: AdvML for LMMs and LMMs for AdvML. This year, in addition to continuing to advance AdvML across the full theory-algorithm-application stack, the workshop is dedicated to addressing the intricate issues that emerge from these converging fields, with a focus on adversarial threats, cross-modal vulnerabilities, defensive strategies, multimodal human/AI feedback, and the overarching implications for security, privacy, and ethics. Join us at AdvML-Frontiers'24 for a comprehensive exploration of adversarial learning at the intersection with cutting-edge multimodal technologies, setting the stage for future advancements in adversarial machine learning. The workshop also hosts the 2024 AdvML Rising Star Award.
AdvML Rising Star Award Announcement
AdvML Rising Star Award was established in 2021 aiming at honoring early-career researchers (senior Ph.D. students and postdoc fellows), who have made significant contributions and research advances in adversarial machine learning. In 2024, the AdvML Rising Star Award will be hosted by AdvML-Frontiers'24 and two researchers are selected and awarded. The awardees will receive certificates and give an oral presentation of their work at the AdvML Frontiers 2024 workshop to showcase their research, share insights, and connect with other researchers in the field. Past Rising Star Awardees can be found at here.
Best Paper Awards
We are pleased to announce the Best Paper Awards for AdvML-Frontiers 2024@NeurIPS 2024:
-
“Can Watermarking Large Language Models Prevent Copyrighted Text Generation and Hide Training Data?”
(Authors: Michael-Andrei Panaitescu-Liess, Zora Che, Bang An, Yuancheng Xu, Pankayaraj Pathmanathan, Souradip Chakraborty, Sicheng Zhu, Tom Goldstein, Furong Huang) -
“Provable Robustness of (Graph) Neural Networks Against Data Poisoning and Backdoor Attacks”
(Authors: Lukas Gosch, Mahalakshmi Sabanayagam, Debarghya Ghoshdastidar, Stephan Günnemann)
Congratulations to these papers!
Past Best Paper Awardees: AdvML-Frontiers'22 and AdvML-Frontiers'23
Keynote Speakers
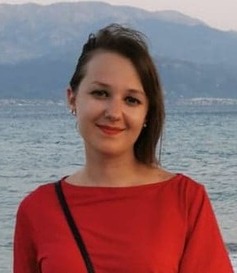
Eleni Triantafillou
Goolge DeepMind, UK
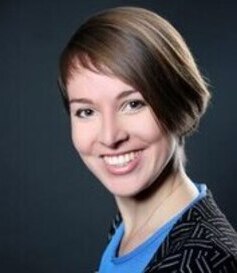
Franziska Boenisch
CISPA Helmholtz Center for Information Security, Germany
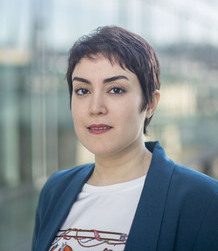
Hoda Heidari
CMU, US
Schedule
Poster Setup
Opening Remarks
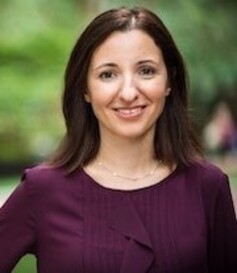
Keynote 1
Alina Oprea
Title: Training Secure Agents: Is Reinforcement Learning Vulnerable to Poisoning Attacks?
Recent advances in Reinforcement learning (RL) have demonstrated how to identify optimal strategies in critical applications such as medical robots, self-driving cars, cyber security defense, and safety alignment in large language models. These applications require strong guarantees on the integrity of the RL methods used for training decision-making agents. In this talk, I will present two recent papers addressing backdoor poisoning attacks on reinforcement learning during the training phase. Both attacks use a novel threat model and significantly improve upon prior RL attacks in the literature in terms of attack success at low poisoning rates, while maintaining high episodic return. Additionally, I will discuss the challenges of designing RL algorithms that are resilient against poisoning attacks.
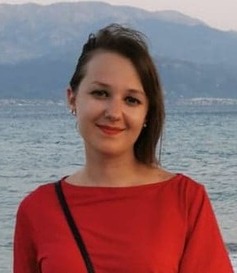
Keynote 2
Eleni Triantafillou
Title: Unlearning memorized data from trained models
Eleni is a senior research scientist at Google DeepMind, based in London. Her main research interest is around creating methods that allow efficient and effective adaptation of deep neural networks to cope with distribution shifts, rapidly learning new tasks, and supporting efficient unlearning of data points.
Training increasingly-large models on increasingly-large datasets poses risks: the resulting models may compromise privacy, may make errors due to poisoned, mislabelled or outdated training data, or pose safety concerns. Unlearning is an umbrella of methods that is designed to remove the effect of certain data points from models after they have already been trained, aiming to avoid the large cost of training models from scratch when unwanted or problematic subsets of their original data are identified. In this talk, I will focus on unlearning memorized training data from models. I will present findings and open questions derived from the first NeurIPS unlearning competition, as well as two pieces of recent work that leverage the tight relationship between memorization and unlearning to derive better unlearning algorithms. I will close with a discussion of important remaining open challenges for future work.
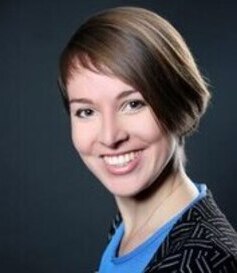
Keynote 3
Franziska Boenisch
Title: From Risks to Resilience: Protecting Privacy in Adapted Language Models
Franziska is a tenure-track faculty at the CISPA Helmholtz Center for Information Security where she co-leads the SprintML lab. Before, she was a Postdoctoral Fellow at the University of Toronto and Vector Institute advised by Prof. Nicolas Papernot. Her current research centers around private and trustworthy machine learning. Franziska obtained her Ph.D. at the Computer Science Department at Freie University Berlin, where she pioneered the notion of individualized privacy in machine learning. During her Ph.D., Franziska was a research associate at the Fraunhofer Institute for Applied and Integrated Security (AISEC), Germany. She received a Fraunhofer TALENTA grant for outstanding female early career researchers, the German Industrial Research Foundation prize for her research on machine learning privacy, and the Fraunhofer ICT Dissertation Award 2023, and was named a GI-Junior Fellow in 2024.
As language models (LLMs) underpin various sensitive applications, preserving privacy of their training data is crucial for their trustworthy deployment. This talk will focus on the privacy of LLM adaptation data. We will see how easily sensitive data can leak from the adaptations, putting privacy in risk. We will then dive into designing protection methods, focusing on how we can obtain privacy guarantees for adaptation data, in particular for prompts. We will also compare private adaptations for open LLMs and their closed, proprietary counterparts across different axes, finding that private adaptations for open LLMs yield higher privacy, better performance, and lower costs. Finally, we will discuss how to monitor privacy of adapted LLMs through dedicated auditing. By identifying privacy risks of adapting LLMs, understanding how to mitigate them, and conducting thorough audits, we can ensure that LLMs can be employed for societal benefits without putting individual data at risk.
Poster Session
(for all accepted papers)
Lunch
Oral Paper Presentation 1
When Do Universal Image Jailbreaks Transfer Between Vision-Language Models?Rylan Schaeffer, Dan Valentine, Luke Bailey, James Chua, Cristobal Eyzaguirre, Zane Durante, Joe Benton, Brando Miranda, Henry Sleight, Tony Tong Wang, John Hughes, Rajashree Agrawal, Mrinank Sharma, Scott Emmons, Sanmi Koyejo, Ethan Perez
Oral Paper Presentation 2
Adversarial Databases Improve Success in Retrieval-based Large Language ModelsSean Wu, Michael Koo, Li Yo Kao, Andy Black, Lesley Blum, Fabien Scalzo, Ira Kurtz
Oral Paper Presentation 3
Provable Robustness of (Graph) Neural Networks Against Data Poisoning and Backdoor AttacksLukas Gosch, Mahalakshmi Sabanayagam, Debarghya Ghoshdastidar, Stephan Günnemann
Oral Paper Presentation 4
Advancing NLP Security by Leveraging LLMs as Adversarial EnginesSudarshan Srinivasan, Maria Mahbub, Amir Sadovnik
Oral Paper Presentation 5
Jailbreak Defense in a Narrow Domain: Failures of Existing Methods and Improving Transcript-Based ClassifiersTony Tong Wang, John Hughes, Henry Sleight, Rylan Schaeffer, Rajashree Agrawal, Fazl Barez, Mrinank Sharma, Jesse Mu, Nir N Shavit, Ethan Perez
Oral Paper Presentation 6
LLM-PIRATE: A Benchmark for Indirect Prompt Injection Attacks in Large Language ModelsAnil Ramakrishna, Jimit Majmudar, Rahul Gupta, Devamanyu Hazarika
Oral Paper Presentation 7
Rethinking Backdoor Detection Evaluation for Language ModelsJun Yan, Wenjie Jacky Mo, Xiang Ren, Robin Jia
Oral Paper Presentation 8
Can Watermarking Large Language Models Prevent Copyrighted Text Generation and Hide Training Data?Michael-Andrei Panaitescu-Liess, Zora Che, Bang An, Yuancheng Xu, Pankayaraj Pathmanathan, Souradip Chakraborty, Sicheng Zhu, Tom Goldstein, Furong Huang
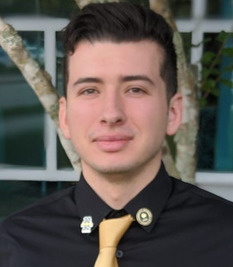
Keynote 4
Alvaro Velasquez
Title: Frontiers in Adversarial AI: Autonomy and Biology
Alvaro Velasquez is a program manager at DARPA, where he currently leads programs on neuro-symbolic and adversarial AI. Before that, Alvaro oversaw the machine intelligence portfolio for the Information Directorate of the Air Force Research Laboratory (AFRL). Alvaro is a recipient of the distinguished paper award from AAAI and best paper and patent awards from AFRL. He has authored over 100 papers and three patents, serves as Associate Editor of the IEEE Transactions on Artificial Intelligence, and is a co-founder of the Neuro-symbolic Systems (NeuS) conference.
Adversarial AI has been instrumental in exposing the vulnerabilities of vision and language systems, particularly in recent years. However, some unconventional domains with great societal implications remain underexplored. In this talk, we briefly discuss two such domains: autonomy and biology. In particular, the sim-to-real gaps in the former present a natural adversary, whereas the latter admits novel threat models with implications on the effectiveness of existing screening tools and the effectiveness of drug discovery.
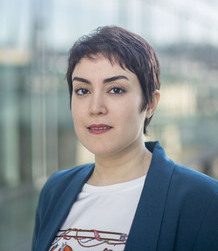
Keynote 5
Hoda Heidari
Title: Red-Teaming for Generative AI: Past, Present, and Future
Hoda Heidari is the K&L Gates Career Development Assistant Professor in Ethics and Computational Technologies at Carnegie Mellon University, with joint appointments in Machine Learning and Societal Computing. She is affiliated with the Human-Computer Interaction Institute and Heinz College of Information Systems and Public Policy. Her research is broadly concerned with the social, ethical, and economic implications of Artificial Intelligence, particularly issues of fairness and accountability through the use of Machine Learning in socially consequential domains. Her work in this area has won a best-paper award at the ACM Conference on Fairness, Accountability, and Transparency (FAccT), an exemplary track award at the ACM Conference on Economics and Computation (EC), and a best-paper award at the IEEE Conference on Secure and Trustworthy Machine Learning (SAT-ML). Dr. Heidari co-founded and co-leads the university-wide Responsible AI Initiative at CMU. She has organized several scholarly events on Responsible and Trustworthy AI, including tutorials and workshops at top-tier Artificial Intelligence academic venues, such as NeurIPS, ICLR, and the Web conference. She is particularly interested in translating research contributions into positive impact on AI policy and practice. She has organized multiple campus-wide events and policy convenings to address AI governance and accountability. Dr. Heidari completed her doctoral studies in Computer and Information Science at the University of Pennsylvania. She holds an M.Sc. degree in Statistics from the Wharton School of Business. Before joining Carnegie Mellon as a faculty member, she was a postdoctoral scholar at the Machine Learning Institute of ETH Zurich, followed by a year at the Artificial Intelligence, Policy, and Practice (AIPP) initiative at Cornell University.
In response to rising concerns surrounding the safety, security, and trustworthiness of Generative AI (GenAI) models, practitioners, and regulators alike have pointed to AI red-teaming as a key component of their strategies for identifying and mitigating these risks. However, significant questions remain about what precisely AI red-teaming entails, what role it can play in risk identification and evaluation, and how it should be conducted to ensure valid, reliable, and actionable results. I will provide an overview of our recent work which analyzes recent cases of red-teaming activities in the AI industry and contrast it with an extensive survey of the relevant research literature to characterize the scope, structure, and criteria for AI red-teaming practices. I will situate our findings in the broader discussions surrounding the evaluation of GenAI and AI governance, and propose an agenda for future work.
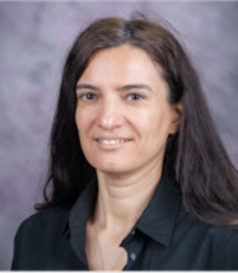
Keynote 6
Cornelia Caragea
Title: Do LLMs Possess Emotional Intelligence?
Cornelia Caragea is a Professor of Computer Science and the Director of the Information Retrieval Research Laboratory at the University of Illinois Chicago (UIC). Caragea currently serves as Program Director at the National Science Foundation. Her research interests are in natural language processing, artificial intelligence, deep learning, machine learning, and information retrieval. Caragea's work has been recognized with several National Science Foundation (NSF) research awards, including the prestigious NSF CAREER award. She has published many research papers in top venues such as ACL, EMNLP, NAACL, ICML, AAAI, and IJCAI and was a program committee member for many such conferences. She reviewed for many journals including Nature, ACM TIST, JAIR, and TACL, served on many NSF review panels, and organized several workshops on scholarly big data. In 2020-21, she received the College of Engineering (COE) Research Award, which is awarded to faculty in the College of Engineering at UIC for excellent research contributions. Caragea was included on an Elsevier list of the top 2% of scientists in their fields for her single-year impact in 2020.
Language models perform well on emotion datasets but it remains unclear whether these models indeed understand emotions expressed in text or simply exploit superficial lexical cues (e.g., emotion words). In this talk, I will discuss capabilities of small and large language models to predict emotions from adversarially created emotion datasets. Our human-annotated adversarial datasets are created by iteratively rephrasing input texts to gradually remove explicit emotion cues (while preserving the semantic similarity and the emotions) until a language model yields incorrect predictions. Our analysis reveals that all models struggle to correctly predict emotions when emotion lexical cues become scarcer and scarcer, but large language models perform better than small pre-trained language models.
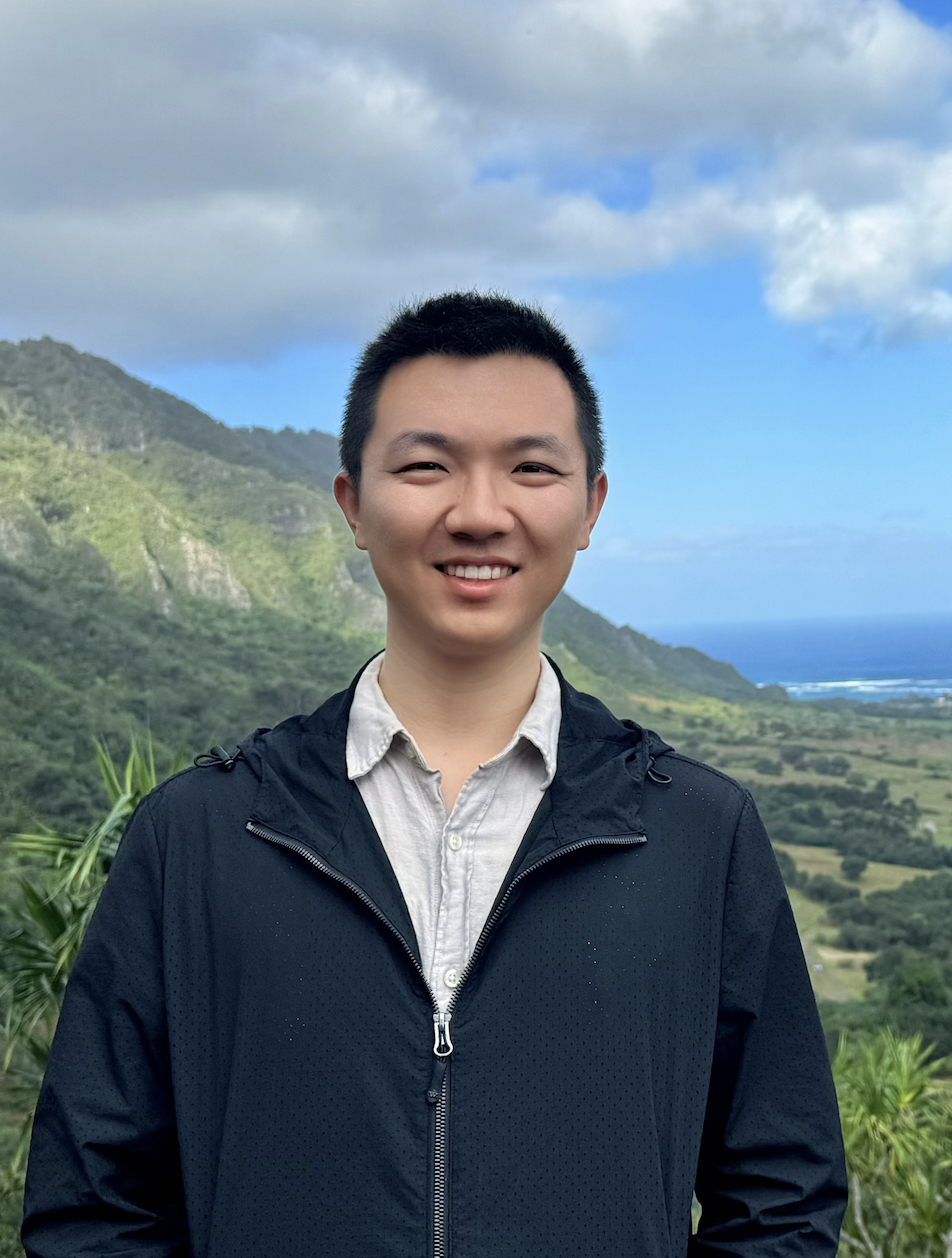
AdvML Rising Star Award Presentation
Awardee: Xuandong Zhao
Title: Empowering Responsible Use of Large Language Models
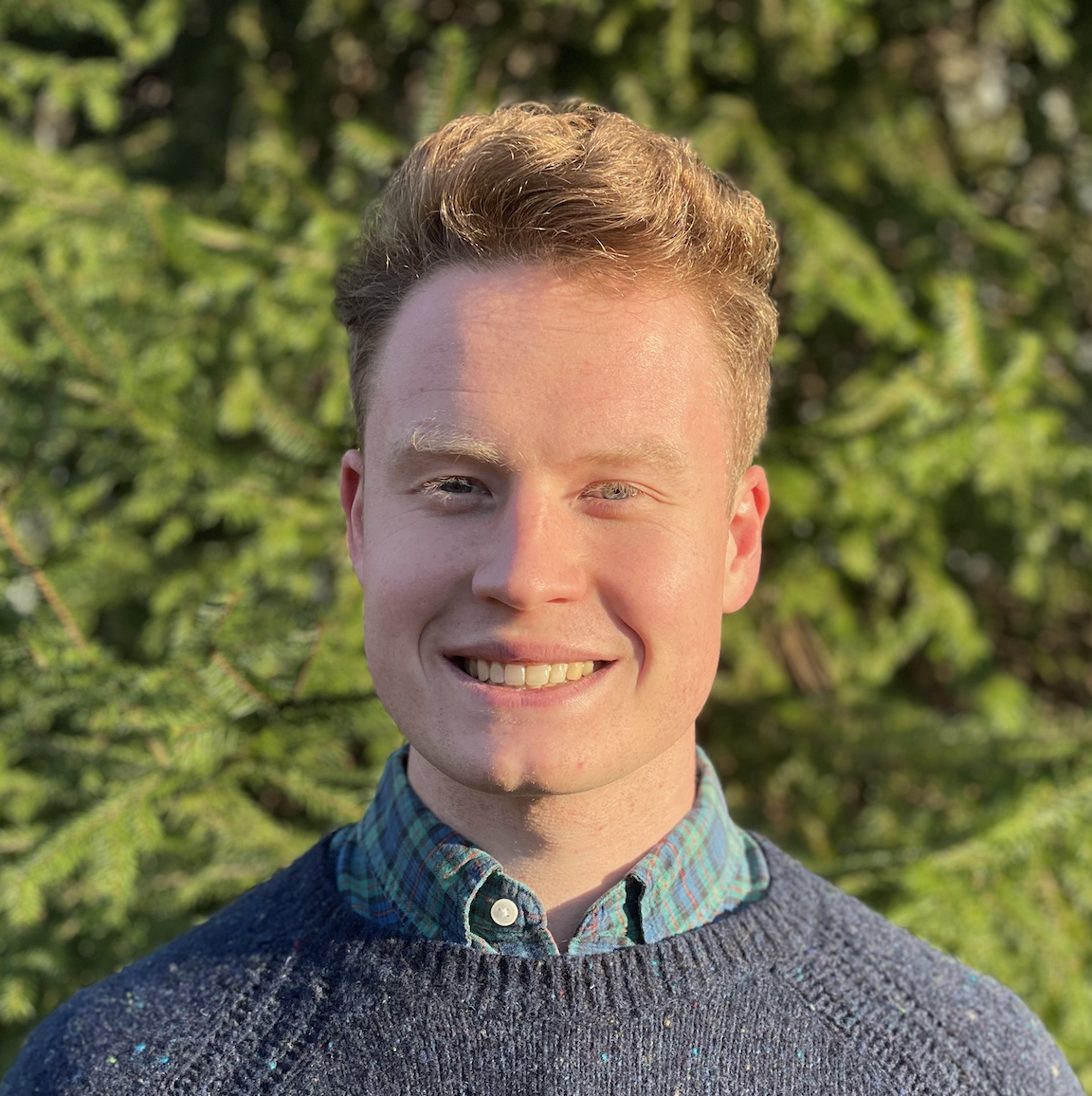
AdvML Rising Star Award Presentation
Awardee: Alexander Robey
Title: Jailbreaking LLM-Controlled Robots
Poster Session and Ending
(for all accepted papers)
AdvML Rising Star Award
Application Instructions
Eligibility and Requirements: Senior PhD students enrolled in a PhD program before December 2021 or researchers holding postdoctoral positions who obtained PhD degree after April 2022.
Applicants are required to submit the following materials:- CV (including a list of publications)
- Research statement (up to 2 pages, single column, excluding reference), including your research accomplishments and future research directions
- A 5-minute video recording for your research summary
- Two letters of recommendation uploaded to this form by the referees before September 9th, 2024 (AoE)
Submission deadline
Application material | Sep 2 '24 AoE | 00:00:00 |
Reference letters | Sep 9 '24 AoE | 00:00:00 |
Call For Papers
Submission Instructions
Submission Tracks
We welcome paper submissions from all the following tracks.
Track 1: Regular paper submission. This track accepts papers up to 6 pages with unlimited references or supplementary materials.
Track 2: Blue Sky Ideas/Position paper submission. This track invites submissions up to 6 pages, focusing on the “future” or “current” directions in AdvML. We welcome papers on visionary ideas, long-term challenges, current debates, and overlooked questions. This track aims to serve as an incubator for innovative and provocative research, providing a platform for the exchange of forward-thinking ideas without the constraints of result-oriented standards.
Track 3: Show-and-Tell Demos submission. This track allows papers up to 6 pages to demonstrate the practical innovations done by research and engineering groups. This track aims to create a unique opportunity to showcase the recent developments in the field through tangible demonstrations of systems, applications, services, and solutions.
Please ensure that all submissions conform to the AdvML-Frontiers'24 format template and please submit to OpenReview. Clearly specify the relevant track number in the title of your submission, for instance, by adding \usepackage[track1]{AdvML_Frontiers_2024} at the start of your main LaTeX document. Note that the track number is for review purposes only and will not be included in the final camera-ready version. The accepted papers are non-archival and non-inproceedings. Concurrent submissions are allowed, but it is the responsibility of the authors to verify compliance with other venues' policies. For NeurIPS, any neurips submissions can be submitted concurrently to workshops. Based on the PC’s recommendation, the accepted papers will be allocated either a spotlight talk or a poster presentation.
Important Dates
Submission deadline | Aug. 30 '24 AoE | 00:00:00 |
Notification to authors | Oct. 9 '24 AoE | 00:00:00 |
Topics
The topics for AdvML-Frontiers'24 include, but are not limited to:
- Adversarial threats on LMMs
- Cross-modal adversarial vulnerabilities for LMMs
- Defensive strategies and adversarial training techniques for LMMs
- Ethical implications of AdvML in LMMs
- Privacy and security in LMMs, (e.g., membership inference attack vs. machine unlearning, watermarking vs. model stealing)
- LMM-aided AdvML (e.g., for attack and defense enhancements)
- Offensive use of LMMs in security
- Novel applications of AdvML for LMMs and LMMs for AdvML
- Mathematical foundations of AdvML (e.g., geometries of learning, causality, information theory)
- Adversarial ML metrics and their interconnections
- New optimization methods for adversarial ML
- Theoretical understanding of adversarial ML
- Data foundations of adversarial ML (e.g., new datasets and new data-driven algorithms)
- Scalable adversarial ML algorithms and implementations
- Adversarial ML in the real world (e.g., physical attacks and lifelong defenses)
- Provably robust machine learning methods and systems
- New adversarial ML applications
- Explainable, transparent, or interpretable ML systems via adversarial learning techniques
- Fairness and bias reduction algorithms in ML
- Adversarial ML for good (e.g., privacy protection, education, healthcare, and scientific discovery)
Official Twitter Account
For all young researchers, the AdvML Rising Star Award deadline is on September 2nd, Deadline for recommendation letters is September 9th. Please share with promising candidates! pic.twitter.com/NtMU5suPB9
— AdvMLFrontiers (@AdvMLFrontiers) July 25, 2024
AdvML-Frontiers 2024 Venue
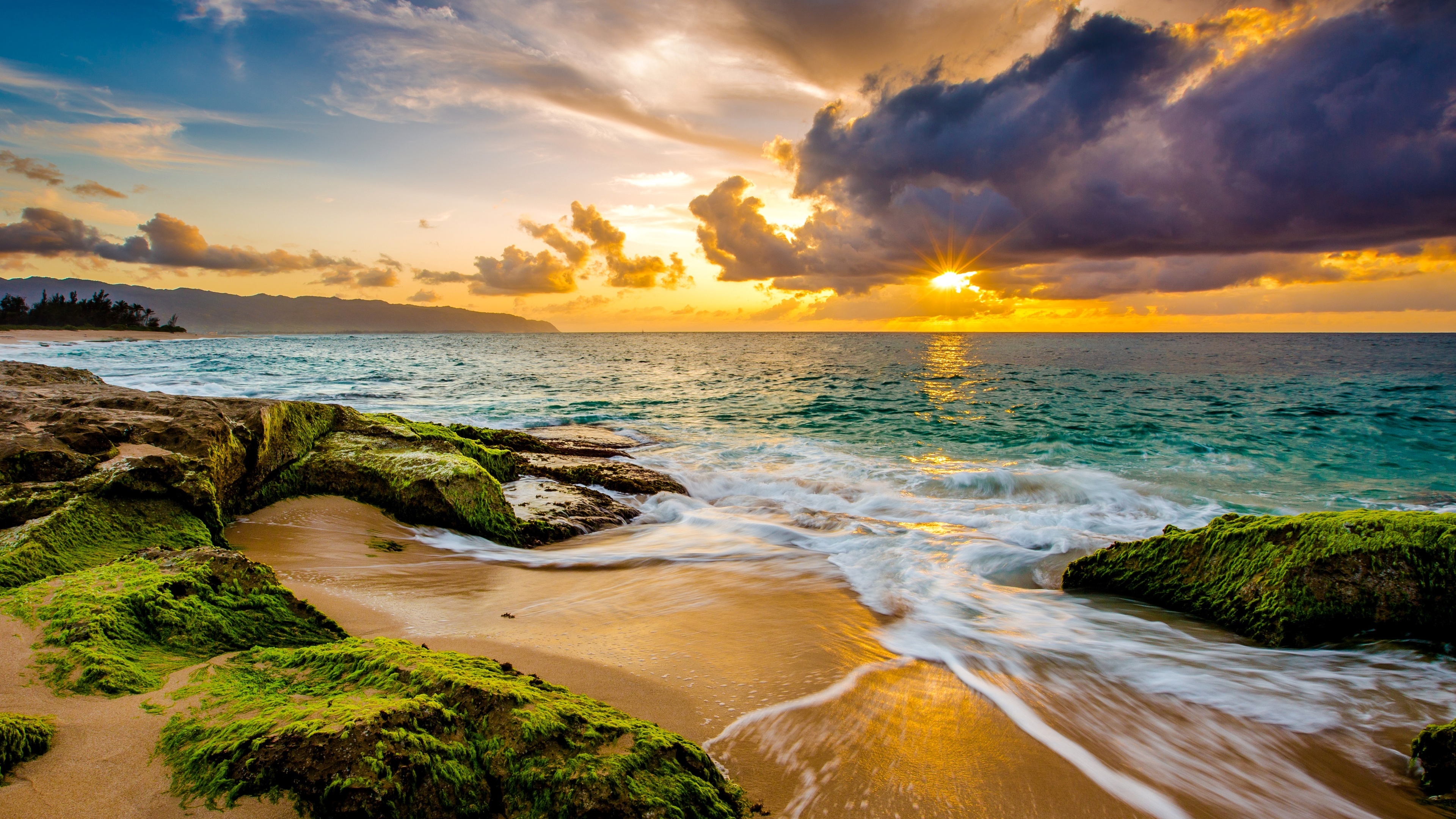
NeurIPS 2024 Workshop
Physical Conference
AdvML-Frontiers'24 will be held in person with possible online components co-located at the NeurIPS 2024 workshop and the conference will take place in the beautiful Vancouver Convention Center, Vancouver, CA.
Organizers
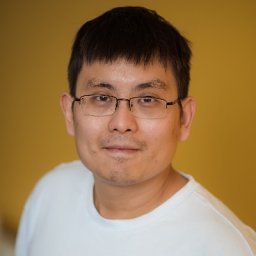
Sijia Liu
Michigan State University, USA
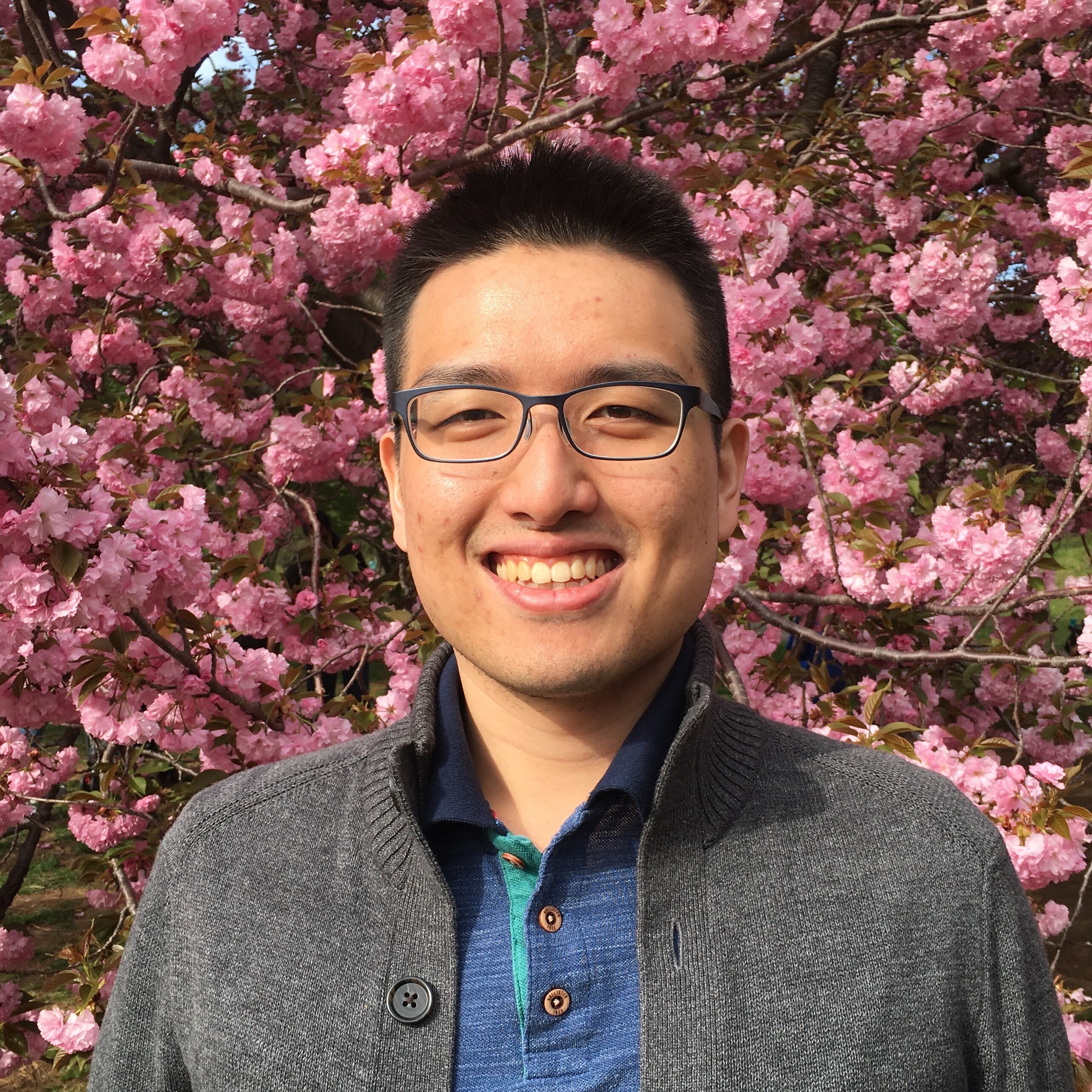
Pin-Yu Chen
IBM Research, USA
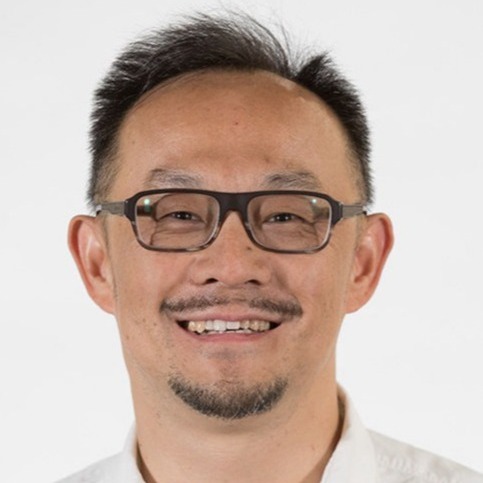
Dongxiao Zhu
Wayne State University, USA
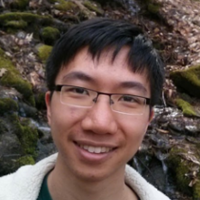
Eric Wong
University of Pennsylvania, USA
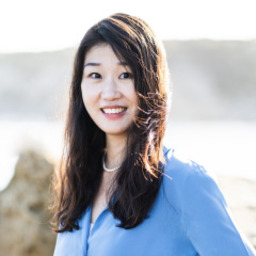
Qin Yao
UC Santa Barbara, USA
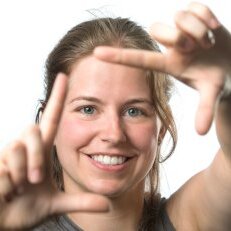
Kathrin Grosse
IBM Research Europe, Switzerland
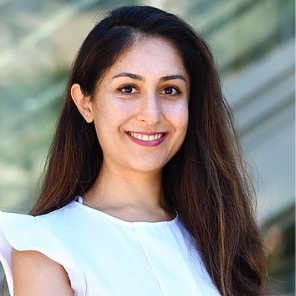
Baharan Mirzasoleiman
UCLA, USA
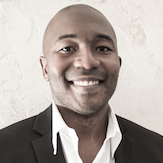
Sanmi Koyejo
Stanford, USA
Program Committee Members
Mathias Humbert (University Lausanne)
Maura Pintor (University of Cagliari)
Maksym Andriuschenko (EPFL)
Yuguang Yao (Michigan State University)
Yiwei Chen (Michigan State University)
Yimeng Zhang (Michigan State University)
Changsheng Wang (Michigan State University)
Soumyadeep Pal (Michigan State University)
Parikshit Ram (IBM Research)
Ruisi Cai (UT Austin (NVIDIA))
Zhenyu Zhang (UT Austin)
Pingzhi Li (UNC)
Haomin Zhuang (Notre Dame)
Changchang Sun (IIT)
Ren Wang (IIT)
Jiabao Ji (UCSB)
Zichen Chen (UCSB)
Deng Pan (University of Notre Dame)
Yao Qiang (Wayne State University)
Rhongho Jang (Wayne State University)
Huaming Chen (The University of Sydney)
Kaiyi Ji (University at Buffalo)
Hossein Hajipour (CISPA)
Siddharth Joshi (UCLA)
Dang Nguyen (UCLA)
Jiayi Ni (UCLA)
Fateme Sheikholeslami (Amazon)
Francesco Croce (EPFL)
Chia-Yi Hsu (NYCU)
Yu-Lin Tsai (NYCU)
Zichong Li (UT Austin)
Zhiyuan He (CUHK)
Shashank Kotyan (Kyushu University)
Zhenhan Huang (RPI)
Wenhan Yang (UCLA)
Jiancheng Liu (Michigan State University)
Chongyu Fan (Michigan State University)
Yuhao Sun (USTC)
Jiaxiang Li (UMN)
Qiucheng Wu (UCSB)
Ioannis Tsaknakis (UMN)
David Pape (CISPA)
Jonathan Evertz (CISPA)
Joel Frank (Ruhr Universität Bochum)
Maximilian Baader (ETH Zurich)
Chirag Agarwal (UVA)
Naman Deep Singh (University of Tubingen)
Christian Schlarmann (University of Tubingen)
Zaitang Li (CUHK)
Chen Xiong (CUHK)
Erh-Chung Chen (NTHU)
Litian Liu (Qualcomm)
Mathilde Raynal (EPFL)
Lena Schoenherr (CISPA)
Yize Li (NEU)
Xin Li (Bosch AI)
Xinlu Zhang (UCSB)
Srishti Gupta (Università di Roma la Sapienza)
Kenan Tang (UCSB)
Prashant Khanduri (Wayne State University)
Ziqi Gao (HKUST)
Aochuan Chen (HKUST)
Junyuan Hong (UT Austin)
Emanuele Ledda (Università di Roma la Sapienza)
Guanhua Zhang (Max Planck Institute for Intelligent Systems)
Giovanni Appruzese (University Liechtenstein)
More to be confirmed ...
Workshop Activity Student Chairs
Yihua Zhang
Yuguang Yao
Changsheng Wang
Contacts
Please contact advml_frontiers24@googlegroups.com for paper submission and logistic questions.