Una-May O'Reilly is the leader of ALFA Group at MIT-CSAIL. An AI and machine learning researcher for 20+ years, she is broadly interested in artificial adversarial intelligence -- the notion that competition has complex dynamics due to learning and adaptation signaled by experiential feedback. This interest directs her to the topic of security where she has develops machine learning algorithms that variously consider the arms races of malware, network and model attacks and the uses of adversarial inputs on deep learning models. Her passions are evolutionary computation and programming. This frequently leads her to investigate Genetic Programming. As well, it draws her to investigations of coevolutionary dynamics between populations of cooperative agents or adversaries, in settings as general as cybersecurity and machine learning.
About AdvML-Frontiers'23
Adversarial machine learning (AdvML), which studies ML in the presence of an adversary, has exhibited great potential to improve the trustworthiness of ML (e.g., robustness, explainability, and fairness), inspire advancements in versatile ML paradigms (e.g., in-context learning and continual learning), and initiate crosscutting research in multidisciplinary areas (e.g., mathematical programming, neurobiology, and human-computer interaction). As the sequel to AdvML-Frontiers’22, AdvML-Frontiers'23 will continue exploring the new frontiers of AdvML in theoretical understanding, scalable algorithm and system designs, and scientific development that transcends traditional disciplinary boundaries. In addition, AdvML-Frontiers’23 will explore trends, challenges, and opportunities of AdvML when facing today's or future large foundational models. Scientifically, we aim to identify the challenges and limitations of current AdvML methods and explore new perspectives and constructive views for next-generation AdvML across the full theory/algorithm/application stack.
AdvML Rising Star Award Announcement
AdvML Rising Star Award was established in 2021 aiming at honoring early-career researchers (senior Ph.D. students and postdoc fellows), who have made significant contributions and research advances in adversarial machine learning. In 2023, the AdvML Rising Star Award will be hosted by AdvML-Frontiers'23 and two researchers are selected and awarded. Congratulations, Dr. Tianlong Chen and Dr. Vikash Sehwag! The awardees will receive certificates and give an oral presentation of their work at the AdvML Frontiers 2023 workshop, providing a platform for them to showcase their research, share insights, and connect with other researchers in the field. Past Rising Star Awardees can be found at here.
Best Paper Awards
The Best Paper Award of Advml-Frontiers'23 has been bestowed upon the paper Adversarial Training Should Be Cast as a Non-Zero-Sum Game! Congratulations to Alexander Robey, Fabian Latorre, George J. Pappas, Hamed Hassani and Volkan Cevher for their outstanding contribution! The award will carry a cash prize with a certificate and the awardees will give an oral presentation of their research in the workshop.
Past Best Paper Awardees: AdvML-Frontiers'22
Accepted Papers
Accepted papers are now available in OpenReview.
Keynote Speakers
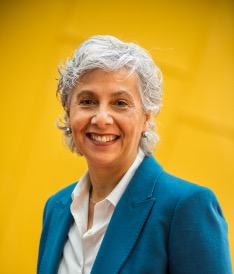
Una-May O’Reilly
Massachusetts Institute of Technology, USA
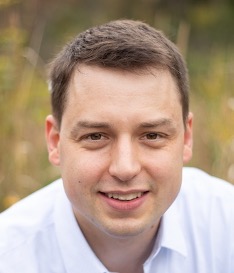
Zico Kolter
CMU, USA
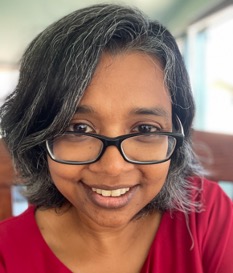
Kamalika Chaudhuri
UCSD, USA
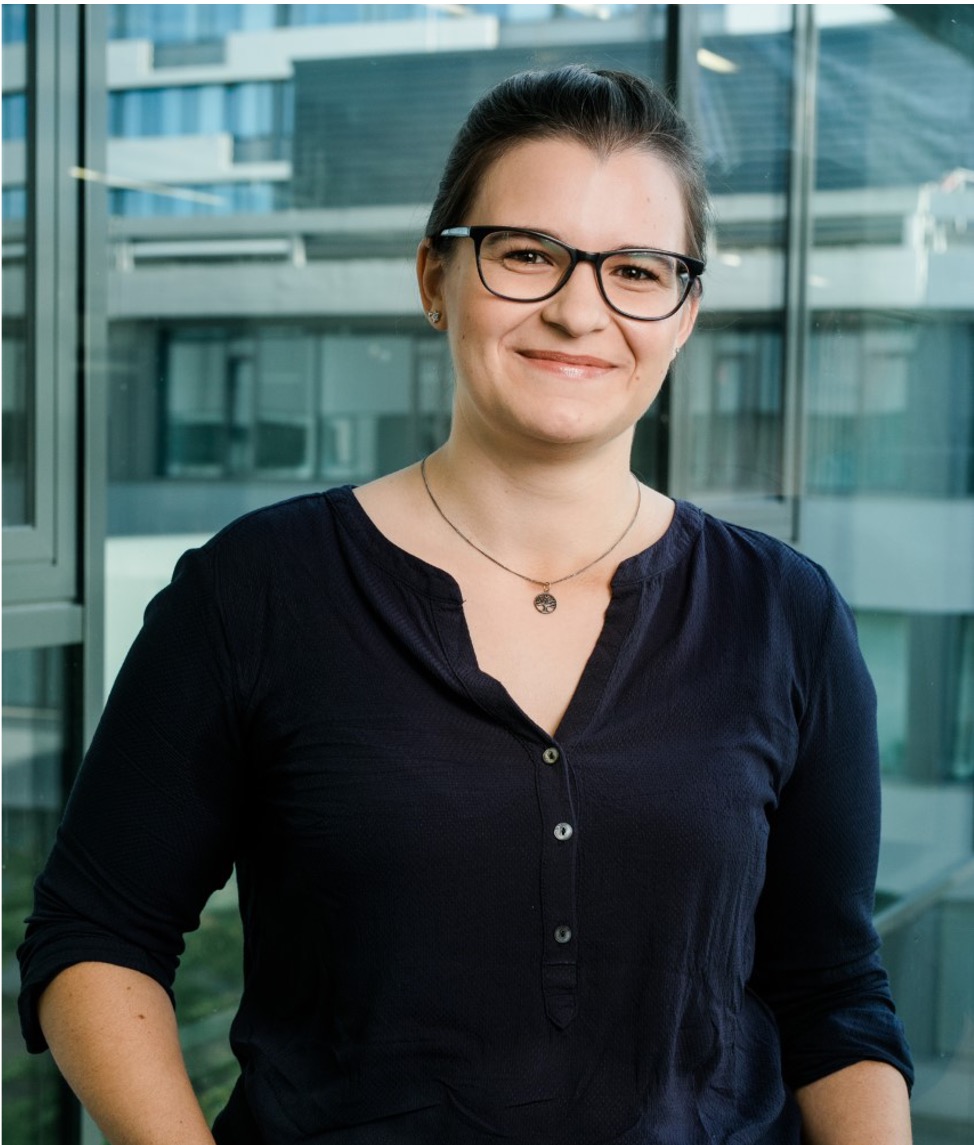
Lea Schönherr
Helmholtz Center, Germany
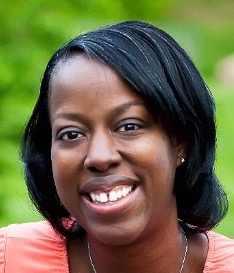
Stacy Hobson
IBM Research, USA
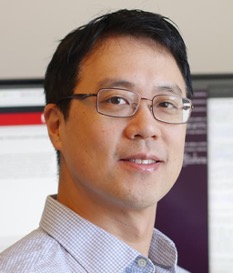
Jihun Hamm
Tulane University, USA
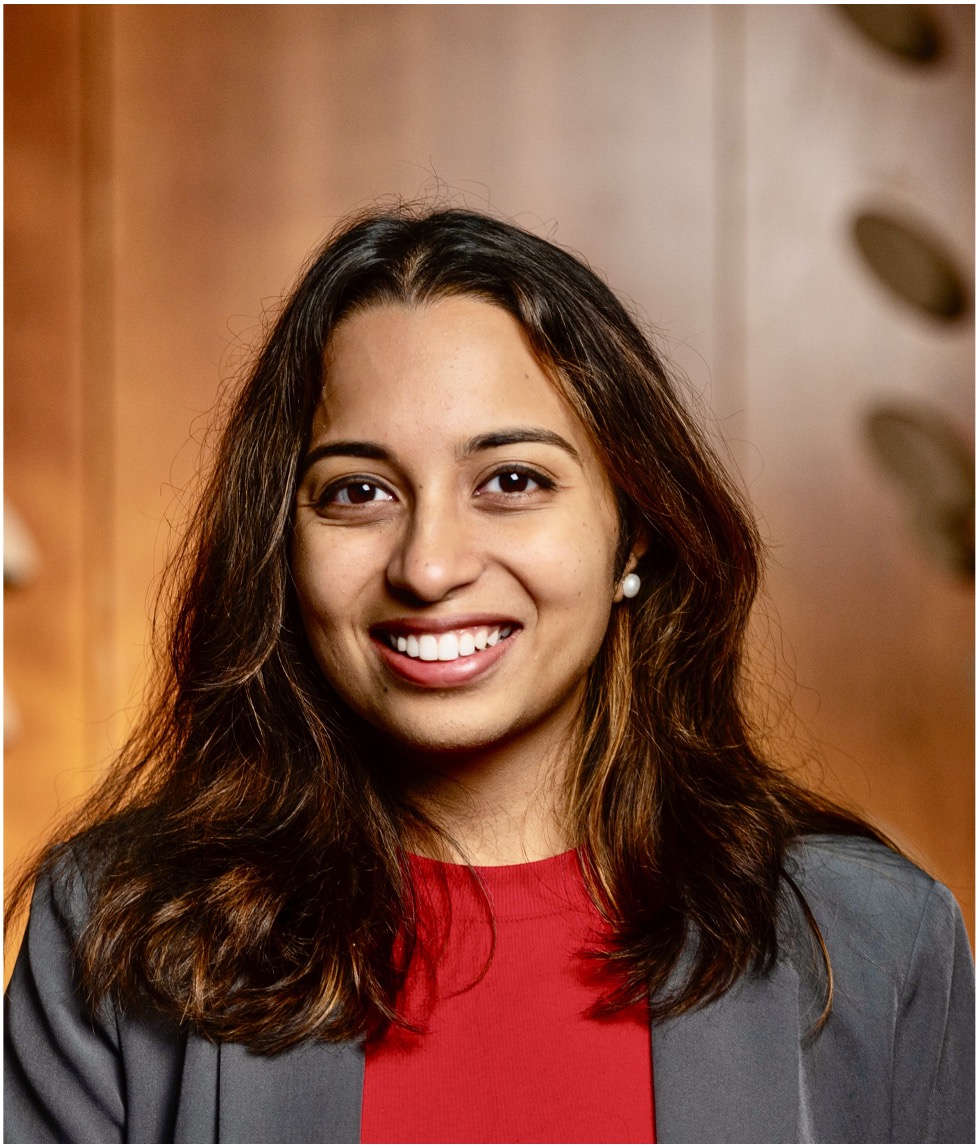
Aditi Raghunathan
CMU, USA
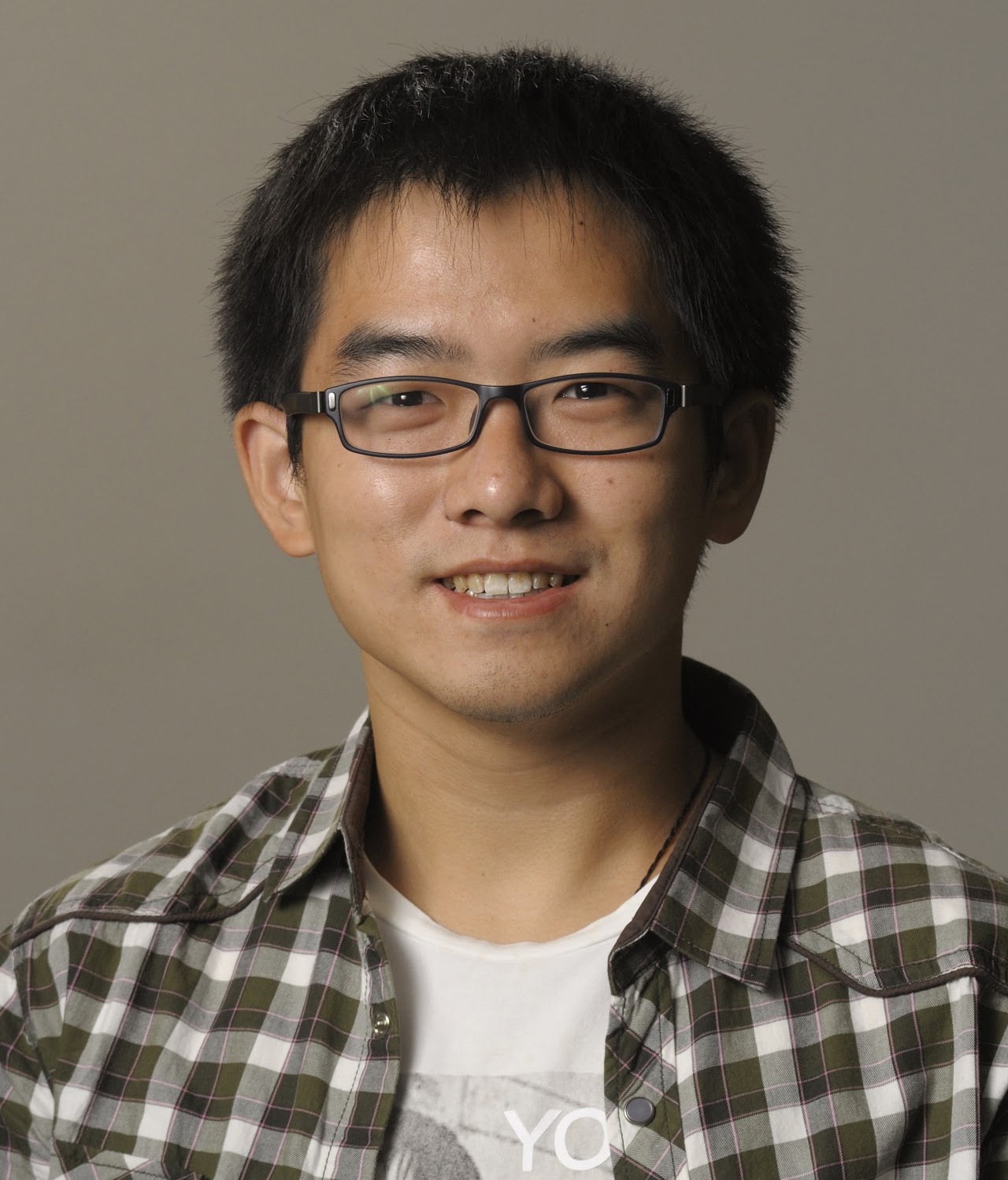
Atlas Wang
UT Austin, USA
Schedule
Opening Remarks
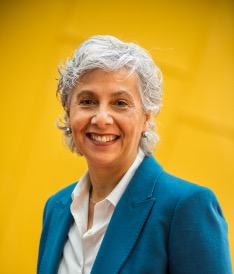
Keynote 1 (Virtual)
Una-May O'Reilly
Title: Adversarial Intelligence Supported by Machine Learning
My interest is in computationally replicating the behavior of adversaries who target algorithms/code/scripts at vulnerable targets and the defenders who try to stop the threats. I typically consider networks as targets but let’s consider the most recent ML models - foundation models. How do goals blur in the current context where the community is trying to simultaneously address their safety and security?
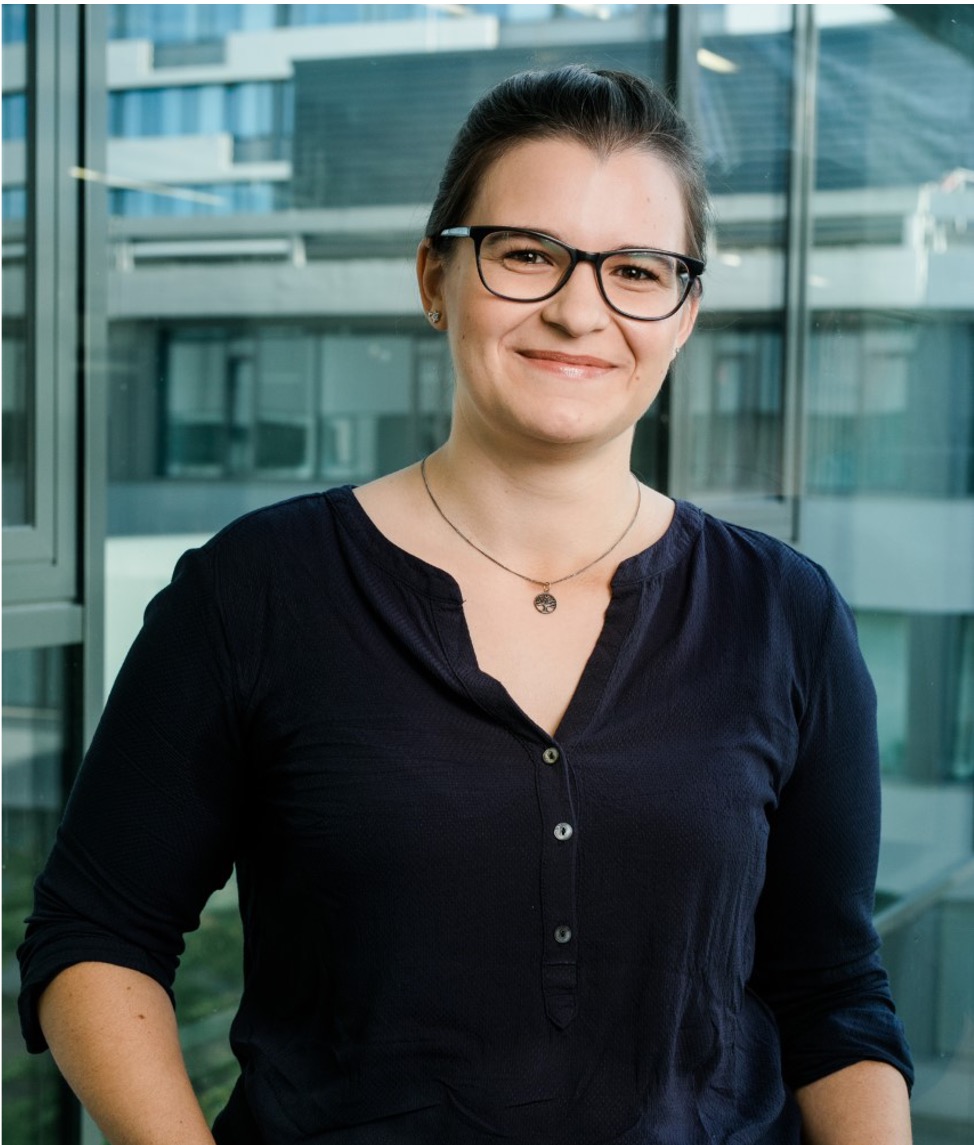
Keynote 2 (Virtual)
Lea Schönherr
Title: Brave New World: Challenges and Threats in Multimodal AI Agent Integrations
Lea Schönherr is a tenure track faculty at CISPA Helmholtz Center for Information Security since 2022. She obtained her PhD from Ruhr-Universität Bochum, Germany, in 2021 and is a recipient of two fellowships from UbiCrypt (DFG Graduate School) and Casa (DFG Cluster of Excellence). Her research interests are in the area of information security with a focus on adversarial machine learning and generative models to defend against real-world threats. She is particularly interested in language as an interface to machine learning models and in combining different domains such as audio, text, and images. She has published several papers on threat detection and defense of speech recognition systems and generative models.
Being on the rise, AI agents become more integrated into our daily lives and will soon be indispensable for countless downstream tasks, be it translation, text enhancing, summarisation or other assisting applications like code generation. As of today, the human-agent interface is no longer limited to plain text and large language models (LLMs) can handle documents, videos, images, audio and more. In addition, the generation of various multimodal outputs is becoming more advanced and realistic in appearance, allowing for more sophisticated communication with AI agents. Particularly in the future, agents will rely on a more natural-feeling voice interface for interactions with AI agents. In this presentation, we will take a closer look at the resulting challenges and security threats associated with integrated multimodal AI agents, which relate to two possible categories: Malicious inputs used to jailbreak LLMs, as well as computer-generated output that is indistinguishable from human-generated content. In the first case, specially designed inputs are used to exploit an LLM or its embedding system, also referred to as prompt hacking. Existing attacks show that content filters of LLMs can be easily bypassed with specific inputs and that private information can be leaked. The use of additional input modalities, such as speech, allows for a much broader potential attack surface that needs to be investigated and protected. In the second case, generative models are utilized to produce fake content that is nearly impossible to distinguish from human-generated content. This fake content is often used for fraudulent and manipulative purposes and impersonation and realistic fake news is already possible using a variety of techniques. As these models continue to evolve, detecting these fraudulent activities will become increasingly difficult, while the attacks themselves will become easier to automate and require less expertise. This creates significant challenges for preventing fraud and the uncontrolled spread of fake news.
Oral Paper Presentation 1
Adversarial Training Should Be Cast as a Non-Zero-Sum GameAlexander Robey, Fabian Latorre, George J. Pappas, Hamed Hassani, Volkan Cevher
Oral Paper Presentation 2
Evading Black-box Classifiers Without Breaking EggsEdoardo Debenedetti, Nicholas Carlini, Florian Tramèr
Oral Paper Presentation 3
Tunable Dual-Objective GANs for Stable TrainingMonica Welfert, Kyle Otstot, Gowtham Raghunath Kurri, Lalitha Sankar
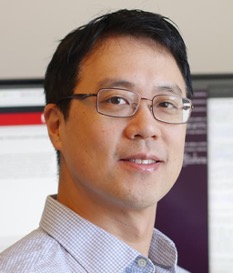
Keynote 3
Jihun Hamm
Title: Analyzing Transfer Learning Bounds through Distributional Robustness
Dr. Jihun Hamm has been an Associate Professor of Computer Science at Tulane University since 2019. He received his PhD degree from the University of Pennsylvania in 2008 supervised by Dr. Daniel Lee. Dr. Hamm's research interest is in machine learning, from theory and to applications. He has worked on the theory and practice of robust learning, adversarial learning, privacy and security, optimization, and deep learning. Dr. Hamm also has a background in biomedical engineering and has worked on machine learning applications in medical data analysis. His work in machine learning has been published in top venues such as ICML, NeurIPS, CVPR, JMLR, and IEEE-TPAMI. His work has also been published in medical research venues such as MICCAI, MedIA, and IEEE-TMI. Among other awards, he has earned the Best Paper Award from MedIA, Finalist for MICCAI Young Scientist Publication Impact Award, and Google Faculty Research Award.
The success of transfer learning at improving performance, especially with the use of large pre-trained models has made transfer learning an essential tool in the machine learning toolbox. However, the conditions under which performance transferability to downstream tasks is possible are not very well understood. In this talk, I will present several approaches to bounding the target-domain classification loss through distribution shift between the source and the target domains. For domain adaptation/generalization problems where the source and the target task are the same, distribution shift as measured by Wasserstein distance is sufficient to predict the loss bound. Furthermore, distributional robustness improves predictability (i.e., low bound) which may come at the price of performance decrease. For transfer learning where the source and the target task are difference, distributions cannot be compared directly. We therefore propose an simple approach that transforms the source distribution (and classifier) by changing the class prior, label, and feature spaces. This allows us to relate the loss of the downstream task (i.e., transferability) to that of the source task. Wasserstein distance again plays an important role in the bound. I will show empirical results using state-of-the-art pre-trained models, and demonstrate how factors such as task relatedness, pretraining method, and model architecture affect transferability.
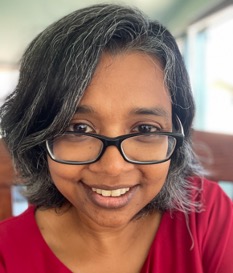
Keynote 4
Kamalika Chaudhuri
Title: Do SSL Models Have Déjà Vu? A Case of Unintended Memorization in Self-supervised Learning
Kamalika Chaudhuri is a Professor in the department of Computer Science and Engineering at University of California San Diego, and a Research Scientist in the FAIR team at Meta AI. Her research interests are in the foundations of trustworthy machine learning, which includes problems such as learning from sensitive data while preserving privacy, learning under sampling bias, and in the presence of an adversary. She is particularly interested in privacy-preserving machine learning, which addresses how to learn good models and predictors from sensitive data, while preserving the privacy of individuals.
Self-supervised learning (SSL) algorithms can produce useful image representations by learning to associate different parts of natural images with one another. However, when taken to the extreme, SSL models can unintendedly memorize specific parts in individual training samples rather than learning semantically meaningful associations. In this work, we perform a systematic study of the unintended memorization of image-specific information in SSL models -- which we refer to as déjà vu memorization. Concretely, we show that given the trained model and a crop of a training image containing only the background (e.g., water, sky, grass), it is possible to infer the foreground object with high accuracy or even visually reconstruct it. Furthermore, we show that déjà vu memorization is common to different SSL algorithms, is exacerbated by certain design choices, and cannot be detected by conventional techniques for evaluating representation quality. Our study of déjà vu memorization reveals previously unknown privacy risks in SSL models, as well as suggests potential practical mitigation strategies.
Poster Session
(for all accepted papers)
Lunch Break + Poster
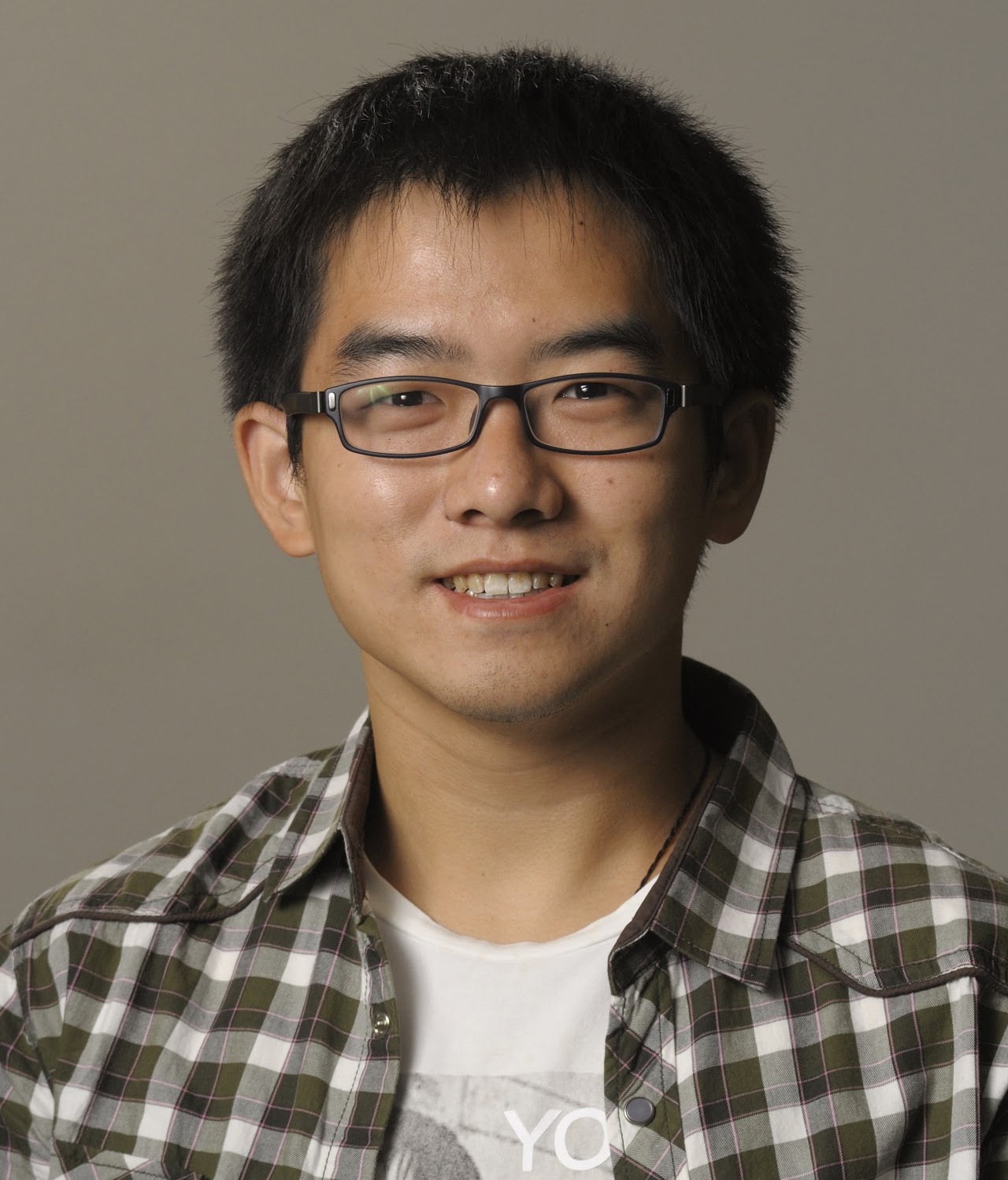
Keynote 5
Atlas Wang
Title: On the Complicate Romance between Sparsity and Robustness
Atlas Wang teaches and researches at UT Austin ECE (primary), CS, and Oden CSEM. He usually declares his research interest as machine learning, but is never too sure what that means concretely. He has won some awards, but is mainly proud of just three things: (1) he has done some (hopefully) thought-invoking and practically meaningful work on sparsity, from inverse problems to deep learning; his recent favorites include “essential sparsity”, “junk DNA hypothesis”, and “heavy-hitter oracle”; (2) he co-founded the Conference on Parsimony and Learning (CPAL), known as the new " conference for sparsity" to its community, and serves as its inaugural program chair; (3) he is fortunate enough to work with a sizable group of world-class students, who are all smarter than himself. He has graduated 10 Ph.D. students that are well placed, including two new assistant professors; and his students have altogether won seven PhD fellowships besides many other honors.
Prior arts have observed that appropriate sparsity (or pruning) can improve the empirical robustness of deep neural networks (NNs). In this talk, I will introduce our recent findings extending this line of research. We have firstly demonstrated that sparsity can be injected into adversarial training, either statically or dynamically, to reduce the robust generalization gap besides significantly saving training and inference FLOPs. We then show that pruning can also improve certified robustness for ReLU-based NNs at scale, under the complete verification setting. Lastly, we theoretically characterize the complicated relationship between neural network sparsity and generalization. It is revealed that, as long as the pruning fraction is below a certain threshold, gradient descent can drive the training loss toward zero and the network exhibits good generalization. Meanwhile, there also exists a large pruning fraction such that while gradient descent is still able to drive the training loss toward zero (by memorizing noise), the generalization performance is no better than random guessing.
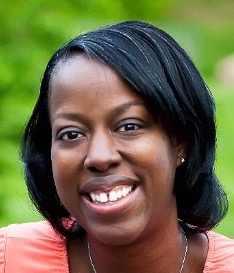
Keynote 6
Stacy Hobson
Title: Addressing technology-mediated social harms
Dr. Stacy Hobson is a Research Scientist at IBM Research and is the Director of the Responsible and Inclusive Technologies research group. Her group’s research focuses on anticipating and understanding the impacts of technology on society and promoting tech practices that minimize harms, biases and other negative outcomes. Stacy’s research has spanned multiple areas including topics such as addressing social inequities through technology, AI transparency, and data sharing platforms for governmental crisis management. Stacy has authored more than 20 peer-reviewed publications and holds 15 US patents. Stacy earned a Bachelor of Science degree in Computer Science from South Carolina State University, a Master of Science degree in Computer Science from Duke University and a PhD in Neuroscience and Cognitive Science from the University of Maryland at College Park.
Many technology efforts focus almost exclusively on the expected benefits that the resulting innovations may provide. Although there has been increased attention in past years on topics such as ethics, privacy, fairness and trust in AI, there still exists a wide gap between the aims of responsible innovation and what is occurring most often in practice. In this talk, I highlight the critical importance of proactively considering technology use in society, with focused attention on societal stakeholders, social impacts and socio-historical context, as the necessary foundation to anticipate and mitigate tech harms.
Oral Paper Presentation 4
Visual Adversarial Examples Jailbreak Aligned Large Language ModelsXiangyu Qi, Kaixuan Huang, Ashwinee Panda, Mengdi Wang, Prateek Mittal
Oral Paper Presentation 5
Learning Shared Safety Constraints from Multi-task DemonstrationsKonwoo Kim, Gokul Swamy, Zuxin Liu, Ding Zhao, Sanjiban Choudhury, Steven Wu
Blue Sky Idea Oral Presentation 1
MLSMM: Machine Learning Security Maturity ModelFelix Viktor Jedrzejewski, Davide Fucci, Oleksandr Adamov
Blue Sky Idea Oral Presentation 2
Deceptive Alignment MonitoringDhruv Bhandarkar Pai, Andres Carranza, Arnuv Tandon, Rylan Schaeffer, Sanmi Koyejo
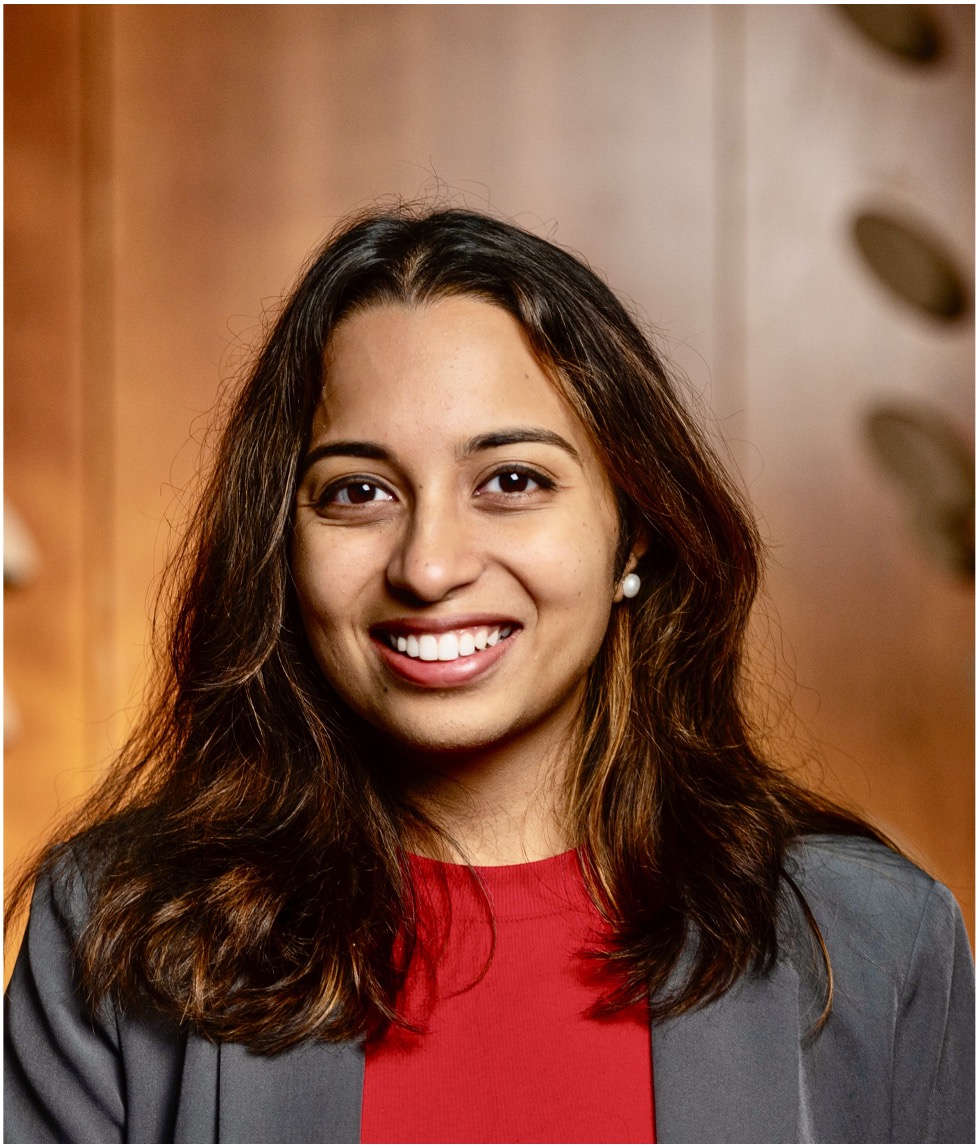
Keynote 7
Aditi Raghunathan
Title: Beyond Adversaries: Robustness to Distribution Shifts in the Wild
Aditi Raghunathan is an Assistant Professor at Carnegie Mellon University. She is interested in building robust ML systems with guarantees for trustworthy real-world deployment. Previously, she was a postdoctoral researcher at Berkeley AI Research, and received her PhD from Stanford University in 2021. Her research has been recognized by the Schmidt AI2050 Early Career Fellowship, the Arthur Samuel Best Thesis Award at Stanford, a Google PhD fellowship in machine learning, and an Open Philanthropy AI fellowship.
Machine learning systems often fail catastrophically under the presence of distribution shift—when the test distribution differs in some systematic way from the training distribution. Such shifts can sometimes be captured via an adversarial threat model, but in many cases, there is no convenient threat model that appropriately captures the “real-world” distribution shift. In this talk, we will first discuss how to measure the robustness to such distribution shifts despite the apparent lack of structure. Next, we discuss how to improve robustness to such shifts. The past few years have seen the rise of large models trained on broad data at scale that can be adapted to several downstream tasks (e.g. BERT, GPT, DALL-E). Via theory and experiments, we will see how such models open up new avenues but also require new techniques for improving robustness.
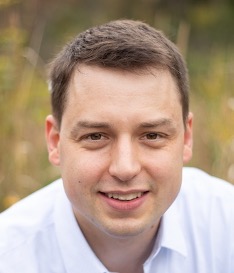
Keynote 8
Zico Kolter
Title: Adversarial Attacks on Aligned LLMs
Zico Kolter is an Associate Professor in the Computer Science Department at Carnegie Mellon University, and also serves as chief scientist of AI research for the Bosch Center for Artificial Intelligence. His work spans the intersection of machine learning and optimization, with a large focus on developing more robust and rigorous methods in deep learning. In addition, he has worked in a number of application areas, highlighted by work on sustainability and smart energy systems. He is a recipient of the DARPA Young Faculty Award, a Sloan Fellowship, and best paper awards at NeurIPS, ICML (honorable mention), AISTATS (test of time), IJCAI, KDD, and PESGM.
In this talk, I'll discuss our recent work on generating adversarial attacks against public LLM tools, such as ChatGPT and Bard. Using combined gradient-based and greedy search on open source LLMs, we find adversarial suffix strings that cause these models to ignore their "safety alignment" and answer potentially harmful user queries. And most surprisingly, we find that these adversarial prompts transfer amazingly well to closed-source, publicly-available models. I'll discuss the methodology and results of this attack, as well as what this may mean for the future of LLM robustness.
Blue Sky Idea Oral Presentation 3
How Can Neuroscience Help Us Build More Robust Deep Neural Networks?Michael Teti, Garrett T. Kenyon, Juston Moore
Blue Sky Idea Oral Presentation 4
The Future of Cyber Systems: Human-AI Reinforcement Learning with Adversarial RobustnessNicole Nichols
Announcement of AdvML Rising Star Award
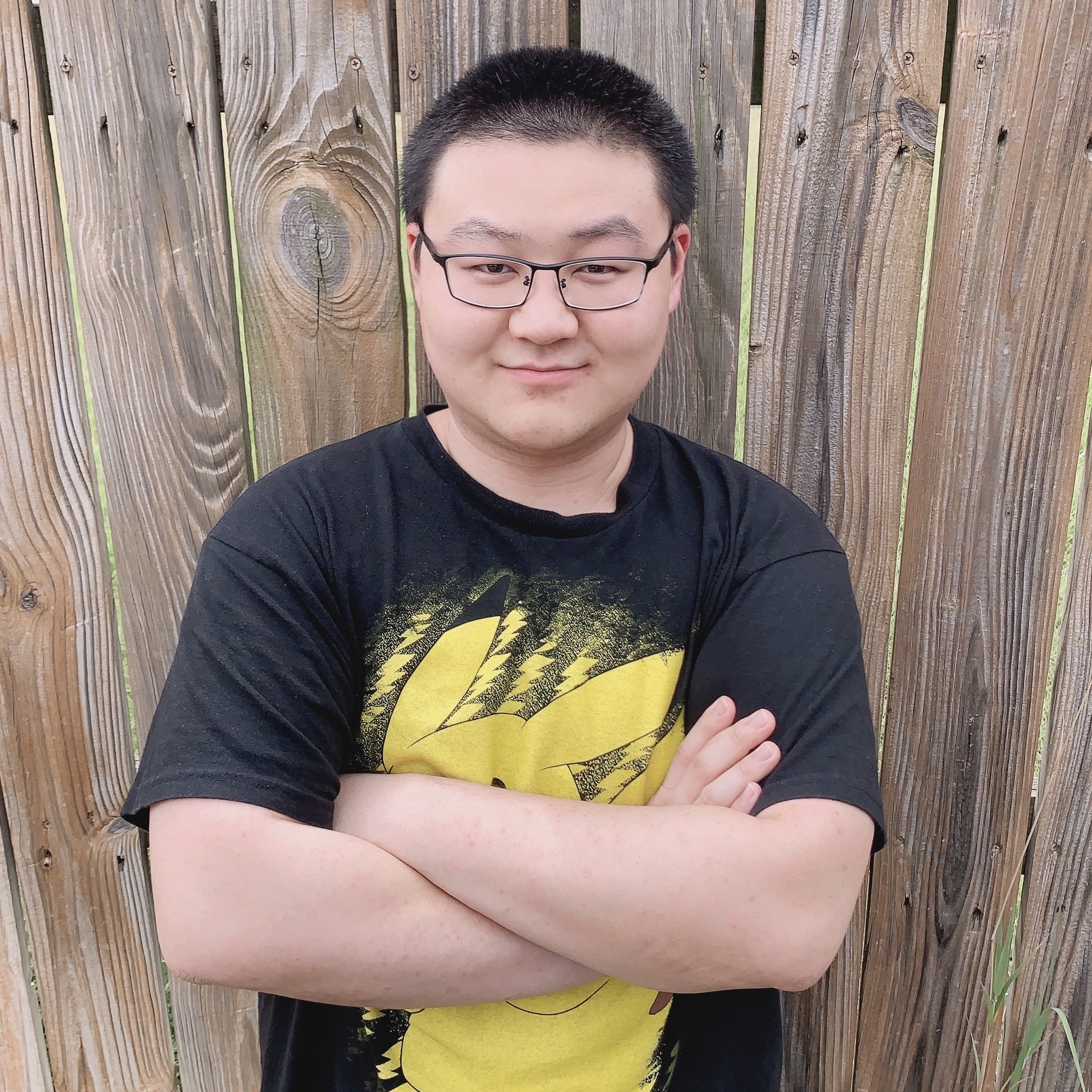
Award Presentation 1
Tianlong Chen
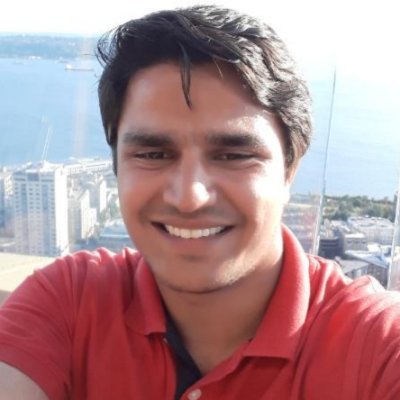
Award Presentation 2
Vikash Sehwag
Poster Session & Closing Remarks
(for all accepted papers)
Closing
Gallery
Check the most exiting moments of Advml-Frontiers'23!
(Photos are taken by Yihua Zhang . If you do not want your photos to be presented here, please contact us!)
AdvML Rising Star Award
Application Instructions
Eligibility and Requirements: Applicants must be senior PhD students who enrolled in a PhD program before December 2020 or researchers holding postdoctoral positions who obtained PhD degree after April 2021.
Applicants are required to submit the following materials:- CV (including a list of publications)
- Research statement (up to 2 pages, single column, excluding reference), including your research accomplishments and future research directions
- A 5-minute video recording for your research summary
- Two reference letters
Submission deadline
Application material | May 26 '23 AoE | 00:00:00 |
Reference letters | Jun 2 '23 AoE | 00:00:00 |
Call For Papers
Submission Instructions
Submission Tracks
We welcome paper submissions from all the following tracks.
Track 1: Full paper reguralar submission. This track accepts papers up to 6 pages with unlimited references or supplementary materials.
Track 2: Blue Sky Ideas submission. This track solicits papers up to 2 pages and targets the future of the research area of adversarial ML. We encourage short submissions focusing on visionary ideas, long-term challenges, and new research opportunities. It serves as an incubator for innovative and provocative ideas and dedicates to providing a forum for presenting and exchanging far-sighted ideas without being constrained by result-oriented standards. We especially encourage ideas on the novel, overlooked, and under-represented areas related to adversarial ML. Selected acceptances in this track will be invited to the Blue Sky Presentation sessions.
Track 3: Show-and-Tell Demos submission. This track allows papers up to 6 pages to demonstrate the innovations done by research and engineering groups in the industry, academia, and government entities.
Submissions for all track should follow the AdvML-Frontiers'23 format template and please submit to OpenReview. The accepted papers are non-archival and non-inproceedings. Concurrent submissions are allowed, but it is the responsibility of the authors to verify compliance with other venues' policies. Based on the PC’s recommendation, the accepted papers will be allocated either a spotlight talk or a poster presentation. All the accepted papers will be publicly available on our workshop website.
Important Dates
Submission deadline | May 28 '23 AoE | 00:00:00 |
Notification to authors | Jun 19 '23 AoE | 00:00:00 |
Camera ready deadline | Jul 21 '23 AoE | 00:00:00 |
Topics
The topics for AdvML-Frontiers'23 include, but are not limited to:
- Mathematical foundations of AdvML (e.g., geometries of learning, causality, information theory)
- Adversarial ML metrics and their interconnections
- Neurobiology-inspired adversarial ML foundations, and others beyond machine-centric design
- New optimization methods for adversarial ML
- Theoretical understanding of adversarial ML
- Data foundations of adversarial ML (e.g., new datasets and new data-driven algorithms)
- Scalable adversarial ML algorithms and implementations
- Adversarial ML in the real world (e.g., physical attacks and lifelong defenses)
- Provably robust machine learning methods and systems
- Robustness certification and property verification techniques
- Generative models and their applications in adversarial ML (e.g., Deepfakes)
- Representation learning, knowledge discovery and model generalizability
- Distributed adversarial ML
- New adversarial ML applications
- Explainable, transparent, or interpretable ML systems via adversarial learning techniques
- Fairness and bias reduction algorithms in ML
- Transfer learning, multi-agent adaptation, self-paced learning
- Risk assessment and risk-aware decision making
- Adversarial ML for good (e.g., privacy protection, education, healthcare, and scientific discovery)
- Adversarial ML for large foundation models.
AdvML-Frontiers 2023 Venue
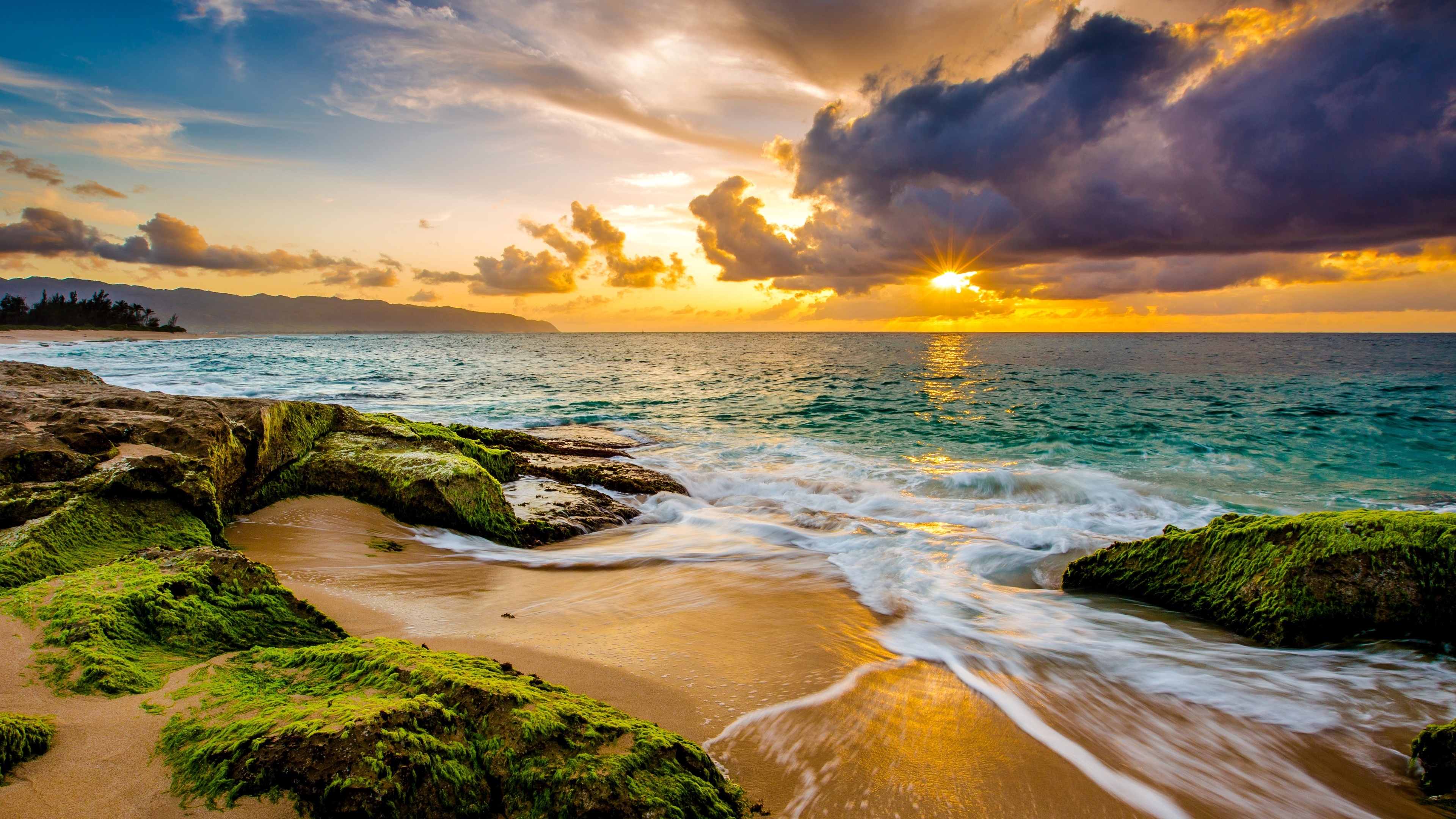
ICML 2023 Workshop
Physical Conference
AdvML-Frontiers'23 will be held in person with possible online components co-located at the ICML 2023 workshop and the conference will take place in the beautiful Honolulu, Hawaii, USA.
Organizers
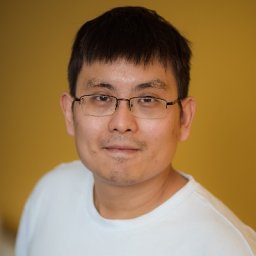
Sijia Liu
Michigan State University, USA
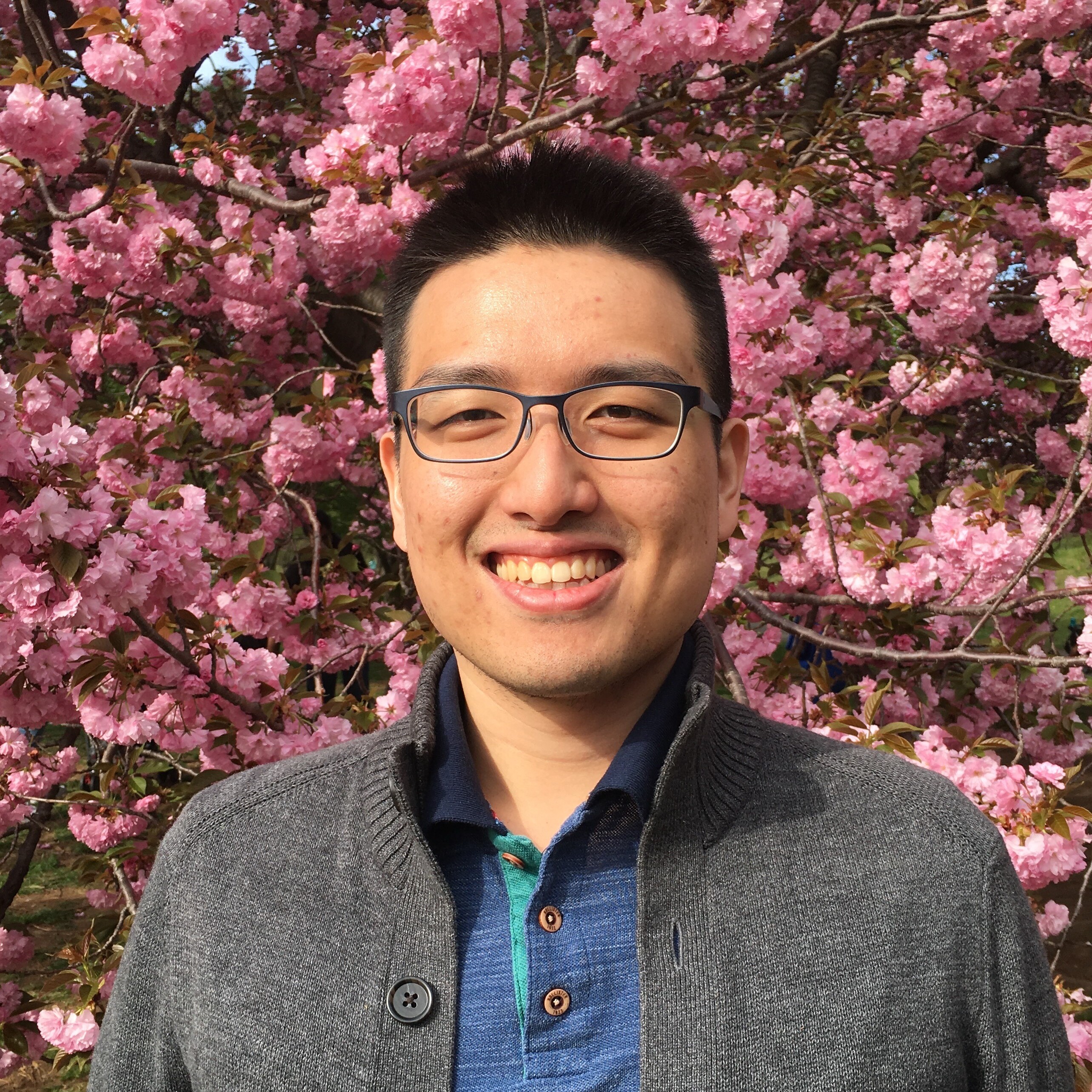
Pin-Yu Chen
IBM Research, USA
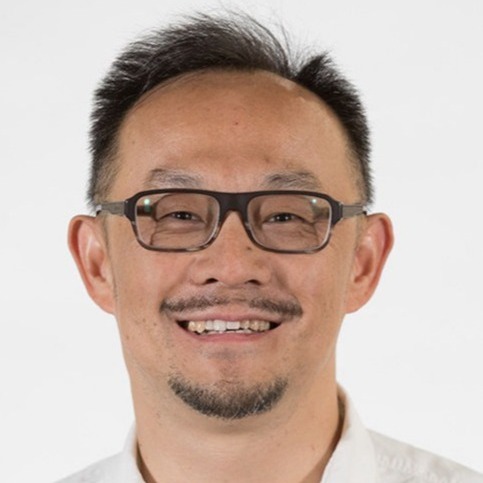
Dongxiao Zhu
Wayne State University, USA
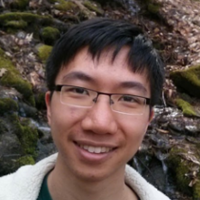
Eric Wong
University of Pennsylvania, USA
Workshop Activity Student Chairs
Yihua Zhang
Yuguang Yao
Contacts
Please contact Yuguang Yao and Yihua Zhang for paper submission and logistic questions. Please contact Dongxiao Zhu, Kathrin Grosse, Pin-Yu Chen, and Sijia Liu for general workshop questions.